Alex Gagliano '17
April 15, 2024
Alex Gagliano
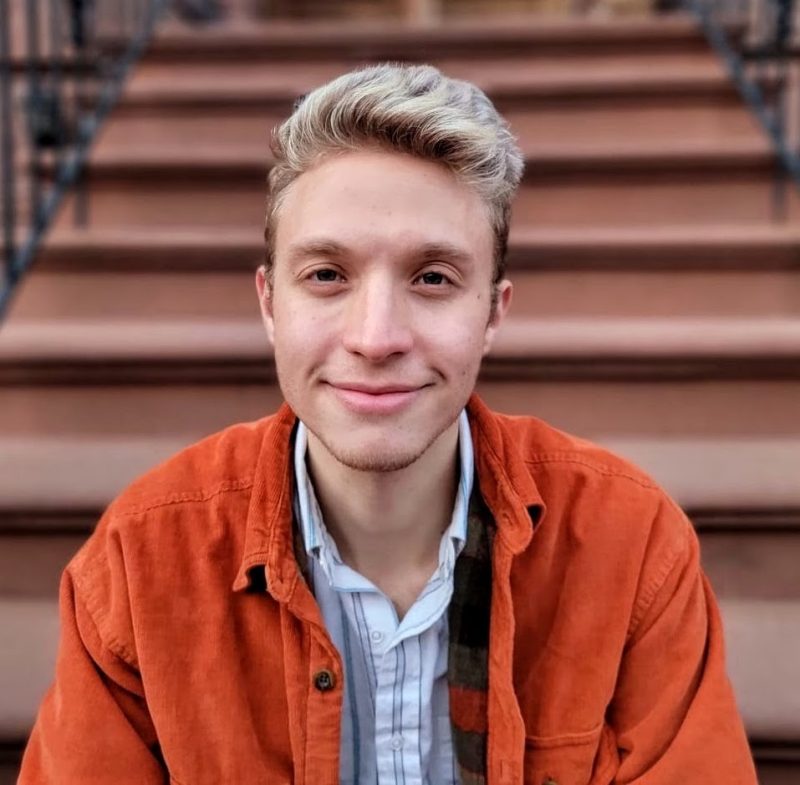
A member of the first Computational Modeling & Data Analytics (CMDA) class at Virginia Tech, Alex Gagliano ’17 is currently a postdoctoral research fellow with the Institute for AI and Fundamental Interactions in Physics, funded by the National Science Foundation. After receiving his undergraduate degree, Gagliano spent a year at the Los Alamos National Laboratory, then moved on to the University of Illinois at Urbana-Champaign, where he earned his Ph.D. in astronomy. In addition to becoming the first known CMDA graduate to receive a doctoral degree, Gagliano co-founded astro[sound]bites, a podcast that discusses cutting-edge research findings in astronomy and highlights the work of early career scientists.
As part of the first CMDA graduating class at Virginia Tech, how did you find out about the program?
I was heavily involved with the Honors College at Virginia Tech. Once I touched down on campus and I was looking for an interdisciplinary major, I wasn't entirely sure what it was going to be. I started in physics and very quickly decided that it just didn't feel right. It wasn't the right fit, and I left. I was working in math for a little while, but I was starting to take some computer science courses, and I was enjoying them a lot.
Anyway, I was applying for a fellowship through the Honors College, and I remember I was at a banquet for the fellowship. There were many different professors there, and we were bouncing around, socializing with everyone. I was commiserating with another undergrad about how we weren't really finding exactly what we wanted at Virginia Tech, and [CMDA director] Mark Embree was within earshot. He came around and offered that they were starting up this new major that was extremely interdisciplinary. It was called computational modeling and data analytics, and it had a little bit of computer science, a little bit of math, a little bit of application in whatever domain you found most interesting. And it sounded like exactly what I wanted to do. I got in as the ideas were still forming about what it would look like, and I'm really glad I did.
Although many CMDA students go straight into industry after graduation, you took the path towards academia. What did that journey look like?
I knew I wanted to go into academia since high school, so to some, the CMDA program might have seemed like a bizarre fit, given that I think a lot of people in industry are thinking about AI, and it's a little more slow-moving in academia. But I was just excited about the problems that people were talking about in CMDA, and I kind of let that guide me.
I did a research program in high school with a scientist at NASA Goddard, and I asked him what advice he had for undergrad if I wanted to eventually become an astrophysicist, and he said take as many math and computer science courses as you can, because astrophysics is a lot of math and computer science. So, I knew that even if a lot of my colleagues were doing something different, that we're headed toward different trajectories, as long as I had a really solid foundation in math and computer science, I would be okay once I left and I found that to be the case. In fact, I found that because a lot of my peers in academia have a more traditional physics background, I lend a unique perspective in being able to spin out models very quickly with insights into the kind of the physical systems that they're interested in studying.
I think it's allowed me to do some pretty unique things. I went to Los Alamos [National Lab] after undergrad for a year and found that to be really fun. Then I went to grad school at the University of Illinois. I did a pre-doc program at the Center for Computational Astrophysics in New York, so it was just a lot of interdisciplinary programs. I feel like I gained the ability to speak to people from many different domains from the CMDA program.
What other strengths did you gain from the CMDA program?
I remember there being a lot of linear algebra, and for a while, I didn't really see how that matched up with the astrophysics courses I was taking through grad school. But now as a postdoc, I'm regularly working with colleagues who are thinking a lot about the theory of machine learning, and how these massive systems train and when you can expect different problems to converge to solutions that you want, and all of that analysis is linear algebra. It’s actually really funny that some of these traditional math and statistical analyses that were being brought up in the CMDA classes are exactly how people are trying to study these deep learning models that we don't know super well how they work. We know that they work well, but we're still trying to figure out why. So, from a technical side, I feel like I actually have a leg up from things that I did my junior year of undergrad with the current research that people are doing as postdocs and professors, so that is astonishing to me.
It’s also just being able to speak to people from many, many different backgrounds. The work that I do now is highly interdisciplinary, and to be able to build machine learning systems, you need to know how they work. You need to know what you want to use them for. You need to know which ones make sense for a given problem. I feel like a lot of that intuition, I picked up in CMDA.
What advice would you give current students in the major about pursuing graduate school?
I would recommend doing research as early as possible. In grad school and beyond, if you stay in academia, research is a massive part of your life and you need to understand early whether you enjoy that sort of rigor, but [also] flexibility. It’s just a different type of lifestyle and it's not for some people, so I would highly recommend getting involved with research as soon as possible.
Also, just explore as many topics as you can in undergrad. If you're following a path that you're excited about, then you tend to hone your skills in a specific area and kind of tunnel in in academia. I think getting that breadth as much as possible in undergrad gives you a leg up in understanding where you want to dig deeper through grad school as a postdoc, as a professor. That’s not to say you can't jump ship later, but it just makes it a lot easier to chart a course that you're excited about.
What are you currently working on?
Right now I'm a postdoc through a fellowship that's funded by the National Science Foundation (NSF). It’s called the Institute for AI and Fundamental Interactions in physics. It’s only a few years old – you can probably see a trend here – and it brings together people from many different fields under the umbrella of machine learning research. There are postdocs in particle physics, in math, and I'm working in astrophysics.
My research relates to the study of how stars explode. I study them observationally and I build machine learning tools to understand their explosions better just from the few sparse observations that we can get. They’re very, very far away, so you only have, in a given image, a single pixel that changes brightness and color over the span of months. And from that changing pixel, you're supposed to infer everything you can about whether the explosion was asymmetric or how energetic the explosion was, what kind of star it came from. So, to be able to do that for many, many different explosions in the sky from just a pixel, automated techniques can get us pretty far. That's a lot of the research that I'm doing now, and in this very diverse center, I'm splitting my time between MIT and Harvard.
What do you think people should know about the CMDA program?
To be fair, it’s been a while since I went through it, so it probably has changed significantly. But what I remember most about it was just how much fun it was.
Work on the problems that you're excited about in undergrad. By far I feel like that has been the biggest thing in undergrad that helped me. The flexibility of CMDA – maybe that's because of CMDA itself, maybe that's because it was so new – but I really got the sense that I could work on anything that I wanted to, and my professors would support me. I could bounce around and find a way to make it work within the given framework that was the curriculum. I just remember having a lot of fun with it, and I think the broad scope of the program allowed for that kind of breadth.
I cannot speak more highly of the program; I just loved my time through it. For a while in early grad school, and even now in academia, I feel like there are some people that are skeptical about how machine learning works and how it can be applied to their given problem, and whether people who do machine learning really know about the underlying physics of the things, the problems that they're trying to solve. But I think if you're having enough fun, it's easy to ignore that people that are skeptical about it, and that's where I'm at. I'm really thankful for CMDA for starting me down that path.