Q&A with ADS Advisory Board Member Oliver Schabenberger
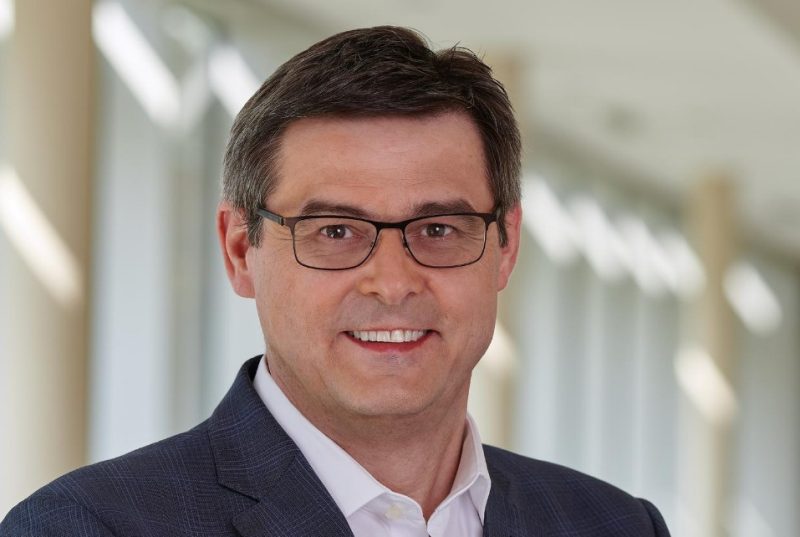
Oliver Schabenberger is a member of the Academy of Data Science Advisory Board and a former CTO, COO, and Chief Innovation Officer. A former associate professor in the Virginia Tech Department of Statistics, Schabenberger is a double Hokie, with a master’s degree in statistics and a Ph.D. in forestry. Here, he discusses the evolution of data science and what the future will bring — and how Virginia Tech can educate successful data scientists.
What has been your experience with data and data science?
Everything in my career has focused around data and analytics. It's been interesting coming into this space as a statistician trained in classical statistical methods, focused on how you think about data and how you express inferences and insights about data.
My approach was statistical modeling. The idea is that a set of data is really generated by some random process, and what you have in front of you — the data, the numbers, and words that you see in the datasets — is one realization of that process. What I really want to learn is — what is that process that generated it? What can I learn about the long run behavior of that process?
When I did my doctorate work at Virginia Tech [in forestry], I’d observe data about the health of forest trees over time. We were trying to understand the probability mechanism that gave rise to the data, because once we understand it, we can then create a model — a statistical model that is an abstraction of that process.
There’s a quote attributed to George E.P. Box that says, “All models are wrong, some are useful,” and that’s sometimes being interpreted as “Don’t use models, they’re all bad.” But that’s not what it means. It means no model wants to be a complete replica of what we're observing — it’s intentionally an abstraction. And if we find that abstraction useful, then we can learn by using the model as a lens and seeing everything through that model. Then we can test hypotheses and make predictions under the model.
That's how I learned how to interact with data. And then over the last two decades, data science entered the field.
How has the rise of data science changed the way people interact with data?
At first it was like — what is this? What is a data scientist and how does this relate to the scientific method? It kind of does not. This idea of making a hypothesis, designing an experiment, collecting data or having observational data, testing the hypothesis against the data — it’s not the prevailing approach [in data science]. It seemed more fluid than the way we were thinking about data. It was driven by what task we want to accomplish, the methods that are available, and then which method works best and how to attune the parameters of those methods.
But they were looking at it from a different lens: one through a model — through a probability mechanism — and one through the idea of a binary target, [like] predicting whether a patient is healthy or not healthy. They seem to sometimes converge on the same techniques from a different perspective, and I thought that was really fascinating. Given those different ways of thinking about data, what do we gain and what do we lose by thinking about data in one way or the other? What I noticed is data science then was taking off quite quickly.
The change came at a time when we were talking about big data in the industry. I never quite understood what big data meant. Is it just large data? I mean, there's something different about working with a dataset of 50 observations and one with 50 million. It takes a different computational technique or different hardware to accomplish a task. But was that the only challenge?
I think big data was a poorly chosen word for the phenomenon that we were experiencing — that the types of data we were working with were different, that the kind of questions we were asking from data were different. The data was moving at a different pace. All of a sudden, there was more unstructured data, textual data. There was data about behavior rather than demography.
We were used to building models on stable variables like age and zip code, and suddenly, our behavior was more expressed by how we use a website or what we clicked on. That behavioral data has a different expiration date. It moves quicker, it changes more frequently, so anything you build with data like this also has a different expiration date. That to me was really what was new.
Computer science started to move from a focus on computing to a focus on data — it discovered data as sort of a focal point of resurgence and investigation. To me, the rise of data science is just the natural evolution of our focus in technology and a focus on research moving to the data — the evolution from industrialization to computerization to datafication.
I think the traditional analytic disciplines — statistics, econometrics, time series, operations research — missed the boat a little bit. They were very much focused on the traditional probabilistic view of data rather than all the new things that you can do with data and that people wanted to do with data. There’s sort of a tension in those two approaches, and I think where the future lies is in bringing the strength of both together.
What are some of the challenges you see in data science today?
The reality of how data science is done in organizations is not necessarily what we want [it] to be. It’s not just about the methods and knowing the best practices of working with data and knowing how to model data — it is about where data science is placed into business today. As so many companies and organizations are trying to become more data-driven, data science takes a very central role. But we’re not doing a good enough job in actually building data science teams and making them successful. Often they are isolated. There are teams that sort of operate in a vacuum — they get data from somewhere, they do clever things, they hand it off and then the models fall on the ground. They’re not being implemented. Data science, to me, in the future, will have a very strategic role in the company.
There is a term for this evolution and this moving from big data to where we are today. I call it data intensity. It is that everything around data is now presenting new challenges. Whether that is a data privacy challenge, a data provenance challenge, a lineage challenge, or just a volume and velocity challenge of data. For example, you build an application that depends on data, and all of a sudden, that application has to manage 10 times as many users as it did before and you can’t scale, or you require too many technologies to get insight from data.
The intensity around data is only going to go up, so what we should think about is how we can train the professionals of the future to deal with that challenge. The winners in the digital economy are those that take advantage of that.
There is data. There's analytics. And then there are decisions. To me, data science is going to evolve into decision science. It’s about the decisions we make. As we become more data-driven, it is going to be increasingly important how we make decisions based on data, how we measure the quality of those decisions, and how we instrument the processes in our business to support decision-making based on data. I think that is the future role of data science.
It's evolving into decision science because more and more of our decisions are based on data.
What do you think is important for future data scientists to learn?
You will have to understand how a finance organization operates within a company, what a marketing organization needs from a data science team, what the core business needs, how data-driven architectures are reflected in products. It's not just, “hey, here's a set of data, let me model this, let me find a well-predicting model or develop a great recommendation engine” — it’s understanding where those things are needed in an organization.I think we should look at the curriculum [of a data science program] from a lens of, how do we build, how do we shape, and how do we educate the leaders of the future? That means it's not just about methodological excellence — it is about understanding how to communicate, how to lead, how to operate in an organization, how to build bridges, how to work across functions. Data science is such essential function in an organization.
How do you actually help digitally transform an organization? A lot of companies are not born digital, but everybody wants to or needs to become more digital. Everybody needs to have more real-time everything — real-time interaction with customers, real-time interactions with employees. What does that mean for how you collect data, how you store data, how you work with data? How do you convince an organization that data scientists work? Those require leadership qualities and the ability to communicate, to speak the language of business. I would hope that the curriculum can reflect that.
I’ve spent 30 years at this — working in academia, working as a software developer building analytic tools, as a leader of R&D organizations all the way up to CTO and COO, and as a leader of innovation teams. Through my experience, I see the gaps that exist in making data science successful. If Virginia Tech can educate graduates that fill those gaps, it'll make the program highly desirable, and the graduates very competitive.
What motivated you to join the advisory board for the Academy of Data Science?
There are a number of factors that played into this. One is that I'm a Virginia Tech alum. I love the university, but really haven’t engaged that much with the university since I graduated.
I’m looking forward to lending a little of my expertise, having been in academia, having been in business. I’ve worked for large companies and am now working for a smaller company. I’ve worked with customers for many years. I’ve walked the walk, from writing my own analytics software to running companies that do that.
I'm also towards the end of my professional career. I think being able to give back — and also to be working with students and passing on some of the learnings and helping them grow — I think that’s something I'm looking forward to doing.
Related information:
Spotlight on VT Alumnus Oliver Schabenberger
NVTC Data Science Speaker Series - From Data Literacy to People-literate Technology: The Reality and Future of Data Science