Data Science Faculty Fellowships
Data Science Faculty Fellowships were established in 2021 by an anonymous donor to enhance the national and international prominence of the study and teaching of data science at Virginia Tech.
The fellowships were established to recognize faculty dedicated to extraordinary research and teaching, to recruit scholars with exceptional records of achievement, and to retain high-performing faculty members with scholarly focus on data science or the application of data science within and across disciplines.
Recipients hold the title of Data Science Faculty Fellow for a period of three years.
2024 Fellows
Yili Hong profile photo
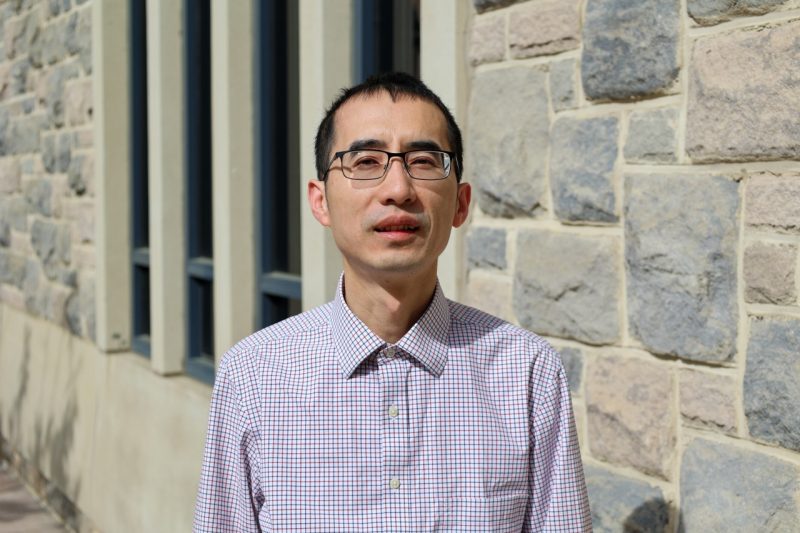
Yili Hong
A member of the Virginia Tech faculty since 2009, Hong serves as professor and co-director of the VT Statistics and Artificial Intelligence Laboratory. Hong’s role in shaping the future of data science is demonstrated in multiple ways with research designed to address three fundamental questions: how to collect data, how to interpret data, and how to use data to predict the future, which are the core tasks at the heart of data science.
His research spans engineering statistics, machine learning/computing, and biostatistics. In engineering statistics, his work emphasizes reliability analysis, particularly the reliability and safety of artificial intelligence systems. In machine learning, he focuses on developing robust algorithms and enhancing computational efficiency. In biostatistics, he investigates survival analysis, longitudinal data analysis, and spatial data analysis.
2023 Fellows
Anne M. Brown profile photo.
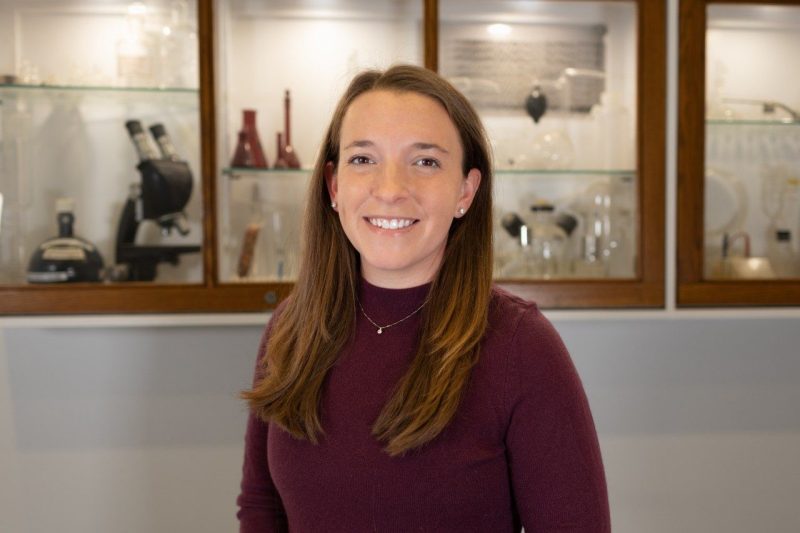
Anne M. Brown
A member of the Virginia Tech faculty since 2016, Brown has continued to focus her research and teaching in the applied data science domain with an emphasis on biomedical and life sciences.
Brown is an associate director within University Libraries Data Services and leads two internationally recognized research programs, Brown Lab and DataBridge. She designs and teaches computational and data-centric life science courses for biochemistry, computational modeling and data analytics (CMDA), and systems biology.
Ivan Hernandez profile photo. Photo by Hunter Gresham.
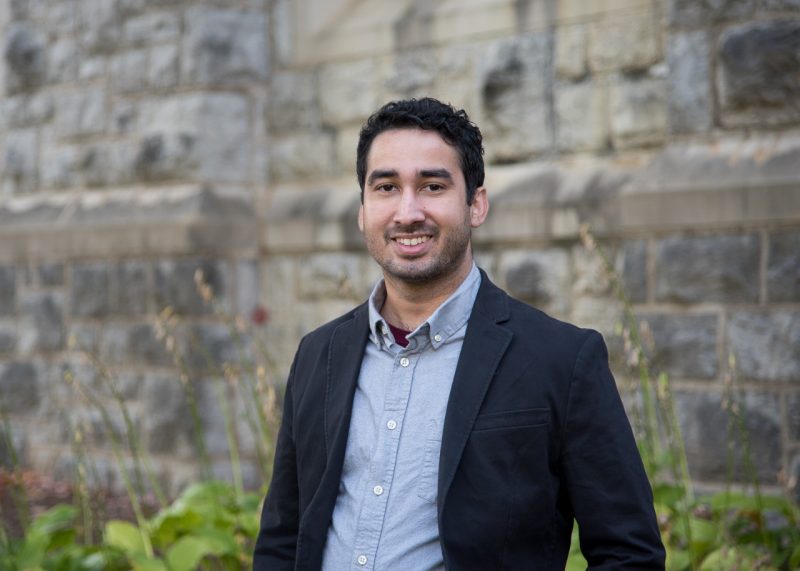
Ivan Hernandez
A member of the Virginia Tech faculty since 2018, Hernandez’s research program is focused on developing new methodologies to facilitate studying organizations and people and incorporates statistical modeling, machine learning, and natural language models. He develops tools and approaches to understand and predict human behavior in group settings, and his work has been published in leading methodological and research journals. He has authored four book chapters and has given more than 50 professional presentations based on his scholarship.
2022 Fellows
Xinwei Deng poses for a photo at Hutchinson Hall
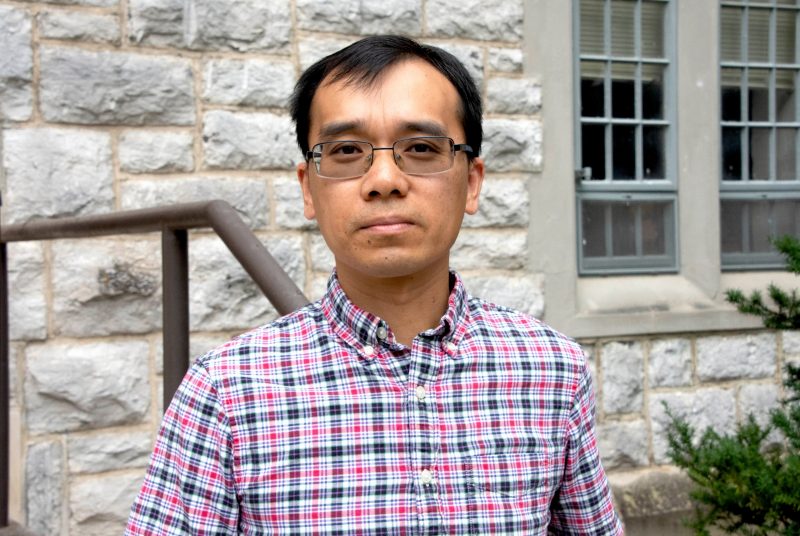
Xinwei Deng
A member of the Virginia Tech faculty since 2011, Xinwei Deng is a data science researcher working with both design of experiments and machine learning for large scale analysis, learning, and decision-making processes. His primary research focuses on developing theoretically sound and computationally efficient methods to model large, complex data; the interface between experimental design and machine learning; and novel statistical methods in emerging areas such as nanotechnology, tissue engineering, environmental science, risk analytics, and epidemiology. His research has practical implications for many important problems facing society.
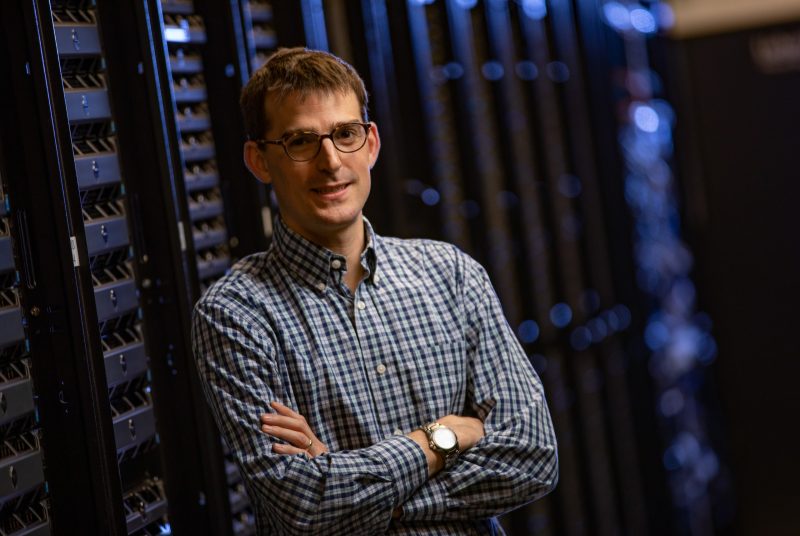
Quinn Thomas
Quinn Thomas has a bold vision: He wants us to predict the natural environment like we currently predict the weather.
“Imagine waking up to a 10-day forecast of the risk of interacting with ticks that have Lyme disease,” said Thomas, an associate professor of forest resources and environmental conservation in the College of Natural Resources and Environment and an associate professor of biology in the College of Science. “Imagine being able to forecast the carbon uptake that a forest is going to collect for a number of years or knowing which reservoirs a resource manager should pull water from and when. And then imagine doing all of that with shared tools, shared frameworks, and a shared computational infrastructure so that learning in one domain can easily transfer to another.”